Research Article
A model of the 2014 Ebola virus: Evidence of West Africa
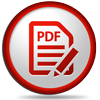
Nadhem Selmi*
Department of Quantitative Methods, Faculty of Economics and Management of Sfax, Tunisia
*Address for Correspondence: Nadhem Selmi, Department of Quantitative Methods, Faculty of Economics and Management of Sfax, Tunisia, Tel: (+216)2170462; Email: [email protected]
Dates: Submitted: 15 April 2019; Approved: 01 May 2019; Published: 02 May 2019
How to cite this article: Selmi N. A model of the 2014 Ebola virus: Evidence of West Africa. Int J Clin Virol. 2019; 3: 010-015. DOI: 10.29328/journal.ijcv.1001004
Copyright License: © 2019 Selmi N. This is an open access article distributed under the Creative Commons Attribution License, which permits unrestricted use, distribution, and reproduction in any medium, provided the original work is properly cited.
Keywords: Ebola 2014; Guinea; SEIR
Abstract
Outbreaks of Ebola virus can cause substantial mortality in affected countries. The largest outbreak of Ebola to date is currently underway in West Africa, with 3944 cases reported as of September 5, 2014. For the sake of deriving a better understanding of the Ebola transmission dynamics, we have undertaken to revisit data from the initial spark of origin of the Ebola virus, which occurred in 1976 in Zaire (now Democratic Republic of Congo). By fitting a mathematical process to time series stratified by disease onset, outcome and source of infection, we have managed to estimate several epidemiological quantities, previously admitted to be too challenging to measure, including hospital and infected community contribution infection to the widespread transmission.
Introduction
The Ebola virus cannot be transmitted through air waves, but rather by close and direct physical contact with infected body fluids: blood, stool and vomiting substances. According to the WHO, traces of the virus may prevail in breast milk, urine and semen. Saliva, tears and sweat represent an extremely low risk. The WHO also indicates that the virus spread through coughing and sneezing is “rare or almost non-existent”. The Ebola virus can also be transmitted during or after contact with surfaces that have been contaminated by infected materials or liquids, such as bed linen and clothes. Health workers may be contaminated by the Ebola virus infected care giving people once if anti-infection measures have not been strictly enforced.
An infected person is not contagious as long as he does not exhibit Ebola disease symptoms. The virus incubation period varies lasts between 2 and 21 days. Still, an infected person remains infectious as the virus is present in blood and body fluids. For instance, the virus may remain in a recovering a man sperm for at least 70 days. In this respect the WHO also recommends the utmost vigilance in case of a direct contact with an infected deceased body.
Noteworthy, the WHO advocates applying strict hygiene measures and risk factors awareness in a bid to restrict Ebola virus transmission to humans. Gloves and personal protection safety equipments are recommended for people dealing with Ebola patients to avoid any contact with body fluids. It is essential to wash hands after paying hospital visits to patients, and after home care provision.
To limit the virus outbreak, the WHO recommends a quick and safe burial of dead corpses, strict identification of issues that may have been in contact with an Ebola infected person, and monitoring such subjects for at least 21 days.
In addition to basic hygiene, special measures need be applied by health care providers on dealing with Ebola infection, such as wearing a respirator, a waterproof gown, a double pair of gloves, goggles, a cap, and waterproof overshoes, all of which should be disposable. Such equipment may also be replaced by a sealed integral overall combination. The patient should wear a disposable pajamas and a surgical mask. If a patient’s case is confirmed, they should be placed in a suitable single-room lacked area. Medical wastes (from care activities for Infectious Risks) must be incinerated.
Understanding AI dynamics in wild bird populations can be made easy by using models that evaluate and integrate diverse scientific data provided by laboratory, experimental and field studies. In addition, disease systems related models can great by help in to assessing the potential effectiveness of alternative management strategies, identifying critical gaps in our knowledge, and determining relative importance of the system different components. An epidemiological SEIR model (Susceptible, Exposed, Infected, and Recovered) is put forward to help examine the Ebola infection related dynamics with respect to individual birds. Ebola viruses are transmitted by bird-to-bird contact or through the surrounding environment. After an infectious contact, a susceptible (S) bird becomes exposed (E) or infected. During the latent period, the AI virus develops within the host until the later becomes infectious (I) by shedding virus. Ebola viruses replicate preferentially in the gastrointestinal tract and are excreted at high levels in the feces. In contrast, recent virus predominant in West Africa and, particularly Ebola viruses isolated since 2002, replicate primarily in wild ducks upper respiratory tract. Ultimately, the host will wither recover (R) or die (D) depending on the interaction between its immune system and the virus (Table 1).
Table 1: Previously published estimates of basic reproduction number, R0, for Ebola. | ||||
Countries | date | R0 | CI 95% | Authors |
DRC | 1995 | 1.83 3.65 2.7 1.38 2.22 1.93 |
3.05-4.33 1.9-2.8 - 1.9-2.73 1.74-2.78 |
Chowell et al. (2004) Ferrari et al. (2005) Legrand et al. (2007) Lekone and Finkenstädt (2006) White and Pagano (2008) Ndanguza et al. (2013) |
Uganda | 2001 | 1.34 1.79 2.7 |
1.52-2.30 2.5-4.1 |
Chowell et al. (2004) Ferrari et al. (2005) Legrand et al. (2007) |
Econometric Methodology
Ebola transmission model
We used a stochastic SEIR type (susceptible-exposed-infectious-recovered) transmission model [1,2], that takes into account the isolation of notified symptomatic individuals and time-dependent transmission rates during different periods of intervention measures put in place during the Ebola virus outbreak on the West Africa described in the main text. Similar compartmental models have been previously developed to study the transmission dynamics of SARS and the 1918 influenza pandemic [3,4].
A figure representation of the individuals’ flow between epidemiological classes is the transmission rate to susceptible S from I; E is the infected (not yet infectious) class of individuals; K is the rate at which E-individuals move to the symptomatic and infectious class I; Infectious individuals (I) would either die or recover at rate γ. I is not an epidemiological state but keeps track of the cumulative number of cases following symptoms’ onset proment.
With respect to our model (Figure 1), the population is classified into five 5 categories of individuals, namely: Susceptible (S), Exposed (E), Symptomatic and infectious (I), Isolated and partially infectious (J), and Recovered (P). Symptomatic infectious individuals are either isolated (category (J)) at the rate α following notification, or recovered without being diagnosed / reported at the rate γ (e.g., unreported mild infections). Isolated individuals are assumed to be partially infectious and the isolation strategy effectiveness is estimated from available data as explained in the following parameter estimation section. The total crew size (N=342) is assumed constant and initially liable to Ebola virus infection. Besides, a well-mixed crew population has also been assumed; every individual has the same probability for having contact with any other within the group. This assumption has been advanced, due in part to the small population setting along with the lack of statistical power necessary for analyzing the relatively small numbers of crew members comprising individual ranks or age groups. Lastly, it has been assumed that previous Ebola vaccination had had no effect on the virus transmission, given the fact that previous data indicate that Ebola vaccines used prior to the 2014 pandemic afforded little protection against Ebola virus [5].
Susceptible crew members infected with the virus have access to the latent period (category E) at the rate β(t)I(t)+lJ(t) / N where β(t) denotes the mean transmission rate per day and at time t and l is stands for the isolated individuals’ relative infectiousness. An initial transmission rate (modeled by parameter β1)) has also been assumed to have remained constant to the coming into effect of the intensive public health measures implemented on March 22th and beginning to be effective for Guinea on March 29th, and changed to β2 during the intervention period (where β2 < β1 if interventions were effective). The ratio (I(t)+lJ(t)) / N represents the probability of having contact with an infectious individual out of the total population sized N. the individual’s latent progress to the infectious and symptomatic class at the rate ( denotes the mean latent period). The mean infectious period is given by . Recovered individuals are assumed protected although the epidemic period duration of the epidemic. The deterministic model applied to describe the above transmission process is given by:
and
where tJuly_5th_intervention stands for the day which the intense public health measures come into effect that is March the 22th. The ordinary differential equation system has been was solved numerically via Matlab (The Mathworks, Inc).
Stochastic simulation of epidemics
It is critically important to consider stochastic rather than deterministic model simulations due to higher stochasticity associated with smaller populations as it is the case with those confined in military ships, for instance. For this sake, stochastic epidemic realizations relevant to the above described model has been derived from the Poisson simulation approach [6], as based on our above indicated deterministic model. Moreover, the outbreak distribution size has also been investigated as a function of the timing and intensity of reactive control interventions.
The reproduction number
The basic reproduction number (R0) is defined as being the number of secondary cases generated by a primary infectious case over its entire infectiousness period within a completely susceptible population and in absence of control interventions [7-10]. When (R0) is greater than one, transmission can occur leading, potentially to an actual epidemic. A major public health interventions’ objective lies in reducing this size to a figure inferior to the soonest possible. A more practical quantity signifies the effective reproduction number R, which accounts for susceptibility changes in the population as an epidemic outbreak unfolds in the population and the control interventions effects. Reproduction figures are useful in determining the interventions’ intensity needed to bring an epidemic under control [2].
Once the epidemiological parameters are estimated, the reproduction number can be estimated by plugging in the estimated parameter values into a formula of the reproduction number. A formula for the reproduction number of our model can be expressed as the sum of the contributions to infection from symptomatic and infectious individuals in the ship that are not yet notified (class I) and those persons that are isolated and are partially infectious (class J).
The contribution of the infectious and not yet notified individuals to the reproduction number is given by:
The contribution of the isolated and partially infectious individuals to the reproduction number is given by:
Hence, the overall reproduction number is then given by:
Parameter estimation
The mean latent period was fixed to 1.5 days and the mean infectious period was bounded in the range 2-3 days according to the epidemiology of Ebola virus [11,12]. The average time from symptoms onset to notification or notification rate (α) was fixed to 1/1.6 days according to the empirical distribution of notification delays of reported cases with laboratory-confirmed Ebola virus on the Guinea and Liberia. To quantify changes in the transmission rate and effectiveness of isolation strategies, a simple epidemic model was fitted to the Ebola virus case epidemic curve by dates of symptom onset by relying on the general approach of “trajectory matching,” where one searches for the combination of model parameters that produces an epidemic curve most statistically similar to the observed one [7,8]. Once epidemiological parameters are estimated, these are used to generate estimates of R using a formula derived from the transmission model.
The transmission rates (β1 and β2), the relative infectiousness of the isolated individuals (l), and the initial numbers of individuals in the exposed E (0) and infectious I (0) categories were estimated by least squares fitting of the model to the daily number of new Ebola virus cases by dates of symptoms onset. Due to the short latent period characteristics of Ebola, [9] we assumed E (0) = I (0); this simplification allowed us to estimate only four parameters from the time series of laboratory-confirmed Ebola virus cases. The reproduction number was estimated using data comprising the epidemic period preceding the start of the intensification of control interventions on March 22th for Guinea and March 29th for Liberia.
Uncertainty analyses
We estimated the uncertainty of the estimated model parameters via parametric bootstrap as in previous studies [4,10]. Briefly, we simulated 100 alternate realizations of the epidemic trajectory, by perturbation of the best-fit curve of daily number of new Ebola cases. We added to the best-fit curve a simulated error structure computed using the increment in the “true” number of cases from day j to day j+1 as the Poisson mean for the number of new cases observed in the j to j+1 interval. The 95% bootstrap-based confidence intervals for the reproduction number should be interpreted as containing 95% of estimates if the analysis was repeated with the same model assumptions and if observational error was the only source of noise.
Results
Our model was able to capture the dynamics of Ebola virus disease, including infections resulting from exposure to contaminated syringes and person-to-person transmission, and the timing of outcomes. By fitting to multiple time series, we were able to jointly estimate a number of key epidemiological parameters (Table 2 and Figure 1). Using these estimates, we calculated R following table 3.
Table 2: Description of the transition rates | |
Transition | Description |
b (t) I(t) + lJ(t) / N | Infection |
(I(t) + lJ(t)) / N | Progression of incubation |
![]() |
Onset of symptoms and infectiousness |
![]() |
Hospitalization and notification |
g | Removal |
Table 3: Estimates of the basic reproduction number, R0, split into different component transmission routes. | ||
Parameters | R estimate (95% CrI) | |
Guinea | Liberia | |
(k-1 = 3 days and ϒ -1 = 3 days) | 0.22 (0.06, 0.23) |
0.07 (0.03,0.42) |
(k-1 = 1.5 days and ϒ -1 = 1.5 days) | 0.31 (0.03, 0.62) |
0.24 (0.05,0.37) |
Estimates and 95% credible intervals of the reproduction number for the Ebola virus outbreak in West Africa based on the sequential Bayesian estimation SEIR method. In this sensitivity analysis, we assume a Negative Binomial distribution for the variance in daily incidence of Ebola virus cases. Estimates are based on epidemiological data prior to the start of control interventions on March 22th, 2014.
Notes on contributors
Nadhem Selmi Ph.D in Qantitatives Methods at the University of Sfax in Tunisia. His primary research interests are in contagion of financial crisis, long memory process, GARCH process and Ebola virus in West Africa.
References
- Diekmann O, Heesterbeek J. Mathematical Epidemiology of Infectious Diseases: Model-building, Analysis, and Interpretation. West Sussex: Wiley; 2000. Ref.: https://tinyurl.com/y59kcjsk
- Anderson RM, RM M. Infectious diseases of humans: Dynamics and Control. Oxford: Oxford University Press; 1991. Ref.: https://tinyurl.com/yyowvjv7
- Chowell G, Fenimore PW, Castillo-Garsow MA, Castillo-Chavez C. SARS outbreaks in Ontario, Hong Kong and Singapore: the role of diagnosis and isolation as a control mechanism. J Theor Biol. 2003; 224: 1-8. Ref.: https://tinyurl.com/y25dusmd
- Chowell G, Ammon CE, Hengartner NW, Hyman JM. Estimation of the reproductive number of the Spanish flu epidemic in Geneva, Switzerland. Vaccine. 2006; 24: 6747-6750. Ref.: https://tinyurl.com/y2bttwzo
- Hancock K, Veguilla V, Lu X, Zhong W, Butler EN, Sun H, et al. Cross-reactive antibody responses to the 2009 pandemic H1N1 influenza virus. N Engl J Med. 2009; 361: 1945-1952. Ref.: https://tinyurl.com/yysh3t8r
- Gustafsson L, Sternad M. Bringing consistency to simulation of population models--Poisson simulation as a bridge between micro and macro simulation. Math Biosci. 2007; 209: 361-385. Ref.: https://tinyurl.com/y5e87pmw
- Chowell G, Ammon CE, Hengartner NW, Hyman JM. Estimating the reproduction number from the initial phase of the Spanish flu pandemic waves in Geneva, Switzerland. Math Biosci Eng. 2007a; 4: 457-470. Ref.: https://tinyurl.com/y6x6bold
- Chowell G, Nishiura H, Bettencourt LM. Comparative estimation of the reproduction number for pandemic influenza from daily case notification data. J R Soc Interface. 2007b; 4: 155-166. Ref.: https://tinyurl.com/y4zeo8xd
- Longini IM, Jr., Halloran ME, Nizam A, Yang Y. Containing pandemic influenza with antiviral agents. Am J Epidemiol. 2004; 159: 623-633. Ref.: https://tinyurl.com/y2nd3pjs
- Chowell G, Miller MA, Viboud C. Seasonal influenza in the United States, France, and Australia: transmission and prospects for control. Epidemiol Infect. 2008; 136: 852-864. Ref.: https://tinyurl.com/yyc2m2zo
- Fraser C, Donnelly CA, Cauchemez S, Hanage WP, Van Kerkhove MD, Hollingsworth TD, et al. Pandemic potential of a strain of influenza A (H1N1): early findings. Science. 2009; 324: 1557-1561. Ref.: https://tinyurl.com/y2ypzwmd
- Cauchemez S, Donnelly CA, Reed C, Ghani AC, Fraser C, Kent CK, et al. Household transmission of 2009 pandemic influenza A (H1N1) virus in the United States. N Engl J Med. 2009; 361: 2619-2627. Ref.: https://tinyurl.com/y4vhlpon